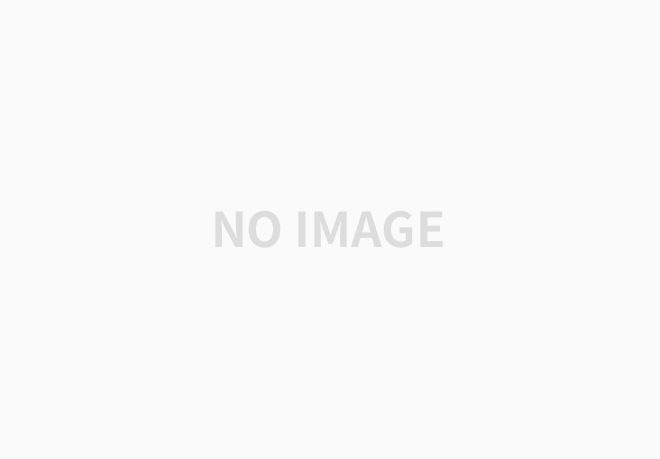
Abstract
The abstract discusses the development of an adaptive approach for precision agriculture monitoring using drone and satellite data. It highlights the importance of precision agriculture for better productivity and food management, emphasizing the use of real-time information from sensors and geospatial techniques to make smarter decisions. The proposed approach involves classifying sparse and dense fields using Landsat 8 satellite data along with drone data, aiming to minimize the repeated usage of drones through an adaptive classification method based on image statistics.
Introduction
The text introduces the importance of precision agriculture in enhancing agricultural productivity by utilizing real-time data from sensors and geospatial technologies like remote sensing and geographic information systems. Precision agriculture involves field-specific crop management practices that enable smarter decision-making for improved productivity. The integration of technologies such as wireless sensor networks, drones, and satellite data plays a crucial role in monitoring agricultural activities at larger scales, aiming to optimize food production and management.
Study fields
The study area for this research is the agriculture fields around Roorkee in Haridwar district, Uttarakhand, India, focusing on sugarcane cultivation. The data used includes multispectral satellite data from Landsat 8 with 11 spectral bands and drone images acquired over the selected agricultural fields. The Landsat 8 data provides detailed information about the study area, while the drone images offer high-resolution visual data for analysis and monitoring purposes in precision agriculture.
What kinds of data used?
In the study, multispectral satellite data from Landsat 8 with 11 spectral bands and a spatial resolution of 30 meters, along with drone images captured using a DJI Phantom quadcopter, were utilized. The Landsat 8 data, acquired from the United States Geological Survey, covered the Haridwar district in Uttarakhand, India, while the drone images were captured over specific agricultural fields in the region. The drone images were mosaicked, orthorectified, and georegistered to provide detailed information for precision agriculture monitoring in the study area.
The way model developed and implemented
In the context of the study, the model development and implementation process focuses on distinguishing between sparse and dense vegetation in large agriculture fields, specifically sugarcane fields. Steps such as cloud masking to remove cloud-affected data, drone image segmentation and gridding to align with Landsat data, vegetation extraction to identify sugarcane areas, ground truth data collection to determine dense vegetation percentage, and band selection for classification are crucial components of this approach. These steps are essential for accurately analyzing and classifying vegetation types within agricultural landscapes using remote sensing data.
Vegetation extraction
In the context of the text, "Vegetation Extraction" refers to the process of identifying and extracting areas of interest, such as sugarcane fields, from images like Landsat data. This involves creating a mask to isolate sugarcane pixels, extracting spectral information for analysis, and collecting ground truth data to determine the percentage of dense vegetation in specific segments. The extracted data is then used to classify areas as either sparse or dense based on predefined criteria.
Threshold apation
Adaptive thresholding is a technique used in precision agriculture monitoring with drones to classify data into sparse and dense vegetation by dynamically adjusting the threshold value based on image statistics. This method overcomes the limitations of fixed thresholding approaches by automatically adapting to spatial and temporal variations in the image data, enhancing the accuracy of vegetation classification without the need for ground truth data for each area of interest. By optimizing the threshold value using performance metrics like overall accuracy and false alarm rate, adaptive thresholding ensures more precise and efficient classification of vegetation types in agricultural fields.
Experiments result
In the results and discussion section, the text explains the implementation results of a methodology involving the classification of sparse and dense vegetation areas using Landsat data and drone images. The methodology includes preprocessing Landsat data, creating a vegetation mask, selecting the appropriate band for classification, and using an adaptive thresholding technique to classify the image into sparse and dense areas with high accuracy, as indicated by producer and user accuracy metrics. Validation of the approach is also discussed by applying it to a different image for accuracy assessment.
Study conclusion
In the conclusion of the study, the researchers discuss the development and implementation of an adaptive approach for monitoring precision agriculture using drones. The approach involves classifying sparse and dense areas within a sugarcane field by combining drone and satellite images, achieving an accuracy of about 87% for testing data and 73% for validation data. The method aims to reduce the need for repetitive drone usage by utilizing image statistics and multiobjective optimization techniques to enhance classification accuracy.